AI in
AV
Download the Solution Brief
Autonomous driving is on the verge
of transforming the transportation industry.
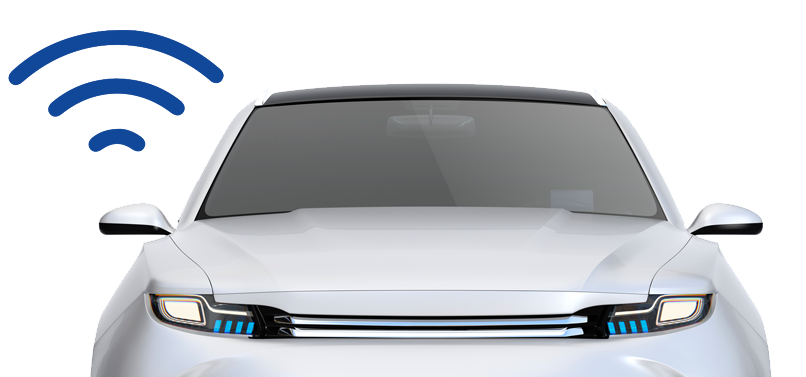
Applications of AI in AV
Challenges
Why is Gaudi a good fit for AV Use Cases?
Customer
Testimonials
“As a world leader in automotive and driving assistance systems, training cutting edge Deep Learning models is mission-critical to Mobileye business and vision. As training such models is time consuming and costly, multiple teams across Mobileye have chosen to use Gaudi-accelerated training machines, either on Amazon EC2 DL1 instances or on-prem; Those teams constantly see significant cost-savings relative to existing GPU-based instances across model types, enabling them to achieve much better Time-To-Market for existing models or training much larger and complex models aimed at exploiting the advantages of the Gaudi architecture. We’re excited to see Gaudi 2’s leap in performance, as our industry depends on the ability to push the boundaries with large-scale high performance deep learning training accelerators.”
Gaby Hayon
Executive Vice President of R&D
Mobileye
“On our own models the increase in price performance met and even exceeded the published 40% mark.”
Chaim Rand
Mobileye
“We are consistently seeing cost-savings compared to existing GPU-based instances across model types, enabling us to achieve much better time-to-market for existing models or training much larger and complex models.”
David Peer
DevOps Tech Lead & Specialist
Mobileye
News & Articles:
- Training On AWS with Habana Gaudi
- Mobileye journey towards scaling Amazon EKS to thousands of nodes leveraging Intel® Xeon® Scalable Processors and Habana’s Gaudi AI accelerators
[1] The Business Research Company, Autonomous Cars Global Market Report 2022, available at https://www.thebusinessresearchcompany.com/report/autonomous-cars-global-market-report